كتاب روابط اجتياز لـ Annex 2. Methods for algorithms for diagnosis of TB in people living with HIV
Algorithms
We modelled the accuracy of four algorithms for the diagnosis of TB in people living with HIV, using WHO-recommended screening and diagnostic tests. Each algorithm was composed of one or more screening tools and one or more confirmatory (diagnostic) tests. Algorithms with more than one screening tool could use parallel screening, in which a positive screen via either tool would prompt confirmatory testing; or sequential screening, in which individuals must screen positive by both tools in order to undergo confirmatory testing. The following assumptions were used for each algorithm:
- All persons in the population will undergo screening and receive a screening result.
- Persons who screen negative will not undergo further evaluation for TB.
- Persons who screen positive will undergo confirmatory testing and receive a test result.
- Persons with a positive confirmatory test result will receive a diagnosis of TB.
- Persons with a negative confirmatory test result will not receive a diagnosis of TB.
- Populations of people with HIV who undergo TB screening
We considered five populations with HIV who would undergo TB screening: 1) people with advanced HIV disease (CD4 cell count <200 cells/μL); 2) people without advanced HIV disease (CD4 cell count >200 cells/μL); 3) outpatients not on ART; 4) outpatients on ART; and 5) inpatients.
Tuberculosis screening tools
We considered the WHO-recommended TB screening tools for people living with HIV (1, 2) comprising the WHO four symptom screen, chest radiography for any abnormality, C-reactive protein with a cut off of >5 mg/L, and molecular WHO-recommended rapid diagnostic tests, drawing from data on Xpert MTB/RIF. Sensitivity and specificity estimates for each screening tool were obtained from two systematic reviews and meta-analyses of TB screening in outpatients (3) and inpatients (4) living with HIV that used data from a WHO-commissioned individual participant data analysis. These reviews informed the WHO consolidated guidelines on tuberculosis. Module 2: screening – systematic screening for tuberculosis disease.
Tuberculosis diagnostic tests
We considered two WHO-recommended TB diagnostic tests for people living with HIV (5, 6), namely, mWRD tests and LF-LAM assays. Although neither test has 100% sensitivity, clinical diagnosis was not considered for those testing negative. Sensitivity and specificity estimates for mWRD were obtained from a systematic review and meta-analysis of the accuracy of Xpert MTB/ RIF in people living with HIV (7) that informed the WHO consolidated guidelines on tuberculosis. Module 3: diagnosis – rapid diagnostics for tuberculosis detection. The incremental yield of LF-LAM over mWRD was estimated based on a literature review (8-11) and was used to estimate the combined sensitivity of LF-LAM and mWRD.
Accuracy of algorithms
Diagnostic accuracy was calculated for each algorithm considering a hypothetical population of 1000 people living with HIV who were screened for TB. The algorithms referenced a range of pretest probabilities of TB from 1% to 30%, taking into account the specifics of each subpopulation. For each algorithm, we calculated the number of true positives, false positives, false negatives, and true negatives detected; the number requiring secondary screening (if applicable); and the number requiring confirmatory (diagnostic) testing. We calculated the net sensitivity and specificity of each algorithm as follows:
- Net sensitivity: the number of true positives detected through confirmatory testing divided by the number of persons with TB disease undergoing screening.
- Net specificity: the number of true negatives detected through confirmatory testing divided by the number of persons without TB disease undergoing screening.
Tables A2.1–A2.4 show the accuracy of the algorithms for the different subgroups within the range of pre-test probabilities.
Costing of the algorithms
Costing was also calculated using a hypothetical population of 1000 people living with HIV who were screened for TB, with a 10% pre-test probability for all algorithms and a 1% pre-test probability of TB for all algorithms, with the exception of Algorithm 4 for medical inpatients with HIV and for people with advanced HIV disease who are seriously ill. Five cost parameters were estimated for each algorithm and population:
- Cost of screening: calculated as the number screened multiplied by the unit cost of the screening tool(s).
- Cost of testing: calculated as the number tested multiplied by the unit cost of mWRD plus the unit costs of LF-LAM.
- Total cost: calculated as the cost of screening plus the cost of testing.
- Cost per TB diagnosis: calculated as the total cost divided by the number of diagnoses of TB disease (true positives and false positives).
- Cost per true TB diagnosis: calculated as the total cost divided by the number of true positive diagnoses of TB disease.
- Cost difference: calculated as the difference in cost for each algorithm, with reference to Algorithm 1.
Unit cost estimates were obtained from the Value TB project, which collected data on costs of TB interventions per episode from 78 health facilities across five countries (Ethiopia, Georgia, India, Kenya and Philippines) (12-16). For these analyses, we utilized median, low, and high unit cost estimates from the countries surveyed. The median cost estimates for the different algorithms to be used among the different subgroups of people living with HIV are provided in Tables A2.5 and A2.6.
Limitations
This analysis has several limitations. First, algorithm accuracy estimates were based on diagnostic accuracy estimates used to inform WHO TB screening and diagnostic guidelines (3, 4, 7). Estimates for the accuracy of mWRD were not available for every population or screening tool, and therefore results may be less precise for some populations. Furthermore, estimates of the incremental yield of LF-LAM over mWRD were limited. Additional systematic reviews of accuracy of mWRD in different HIV populations and the incremental yield of LF-LAM over mWRD would help improve algorithm estimates.
Second, algorithm estimates assumed that all individuals who screen positive will undergo mWRD testing and receive a result. However, in practice, many individuals do not receive a test result, for a variety of reasons. Providers may not refer all individuals who screen positive for confirmatory testing, and some individuals who are referred may not present for testing due to barriers to seeking care. Furthermore, many individuals living with HIV have challenges producing sputum, substantially affecting the yield of sputum-based tests; in addition, the accuracy and yield of sputum-reliant tests is affected by sputum quality. Given these limitations, the true yield of the algorithms is likely lower than the estimates presented here. Importantly, the incremental yield of urine LF-LAM will be higher in populations with low sputum mWRD diagnostic yield (17). Future analyses using diagnostic yield estimates rather than diagnostic accuracy estimates may be useful for TB and HIV programmes developing recommendations for TB screening and diagnostic algorithms for people living with HIV.
Third, costing estimates are based on data from a survey of five countries. Unit costs vary widely globally, and the costs used for analyses here may not be representative of costs in many settings. However, estimates presented here are useful to compare relative differences across algorithms and populations. In addition, cost estimates do not include costs associated with missed episodes of TB, treatment-associated costs or broader health system costs. In practice, algorithms with lower sensitivity may incur costs associated with management of missed TB, while algorithms with higher sensitivity may be associated with higher costs related to treatment of those diagnosed with TB.
Conclusion
Screening and diagnosis of TB in people with HIV is a global health priority, yet there are notable challenges in implementing effective, high-yield, and cost-effective screening and diagnostic algorithms in health-care settings worldwide. In this analysis, we estimated the accuracy and costs of four algorithms for TB screening and diagnosis in people with HIV. Accuracy estimates varied across algorithms, and within algorithms between the populations undergoing screening. In addition, costs varied substantially, and were affected by both cost and accuracy of the screening tool used in each algorithm. HIV and TB programmes should consider the populations being targeted for screening, level of health-care system where screening will be conducted, and resource availability when making local recommendations for TB screening and diagnosis for people with HIV.
RESULTS TABLES
Table A2.1. Algorithm 1
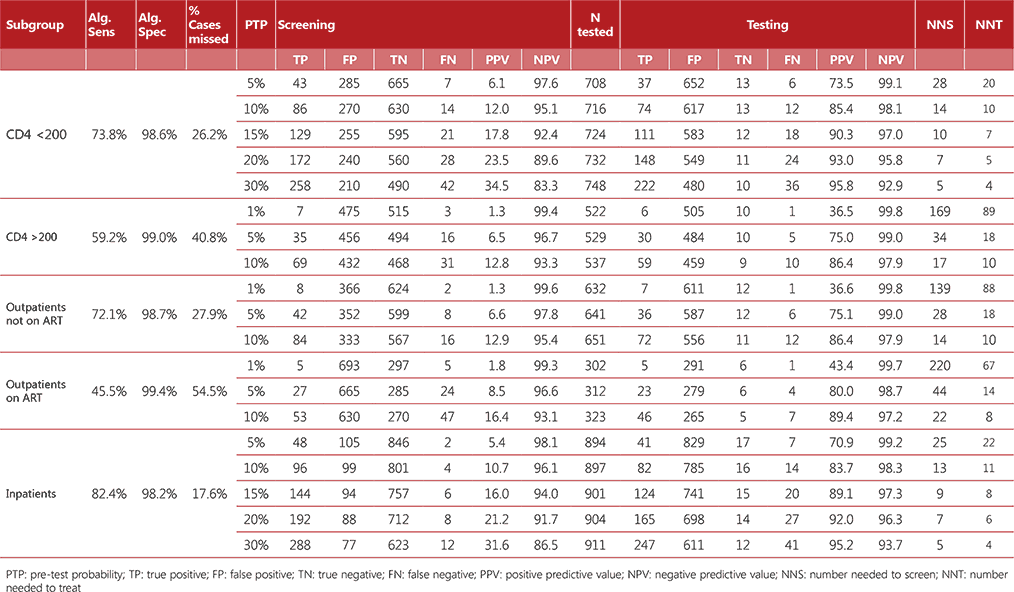
Table A2.2 Algorithm 2
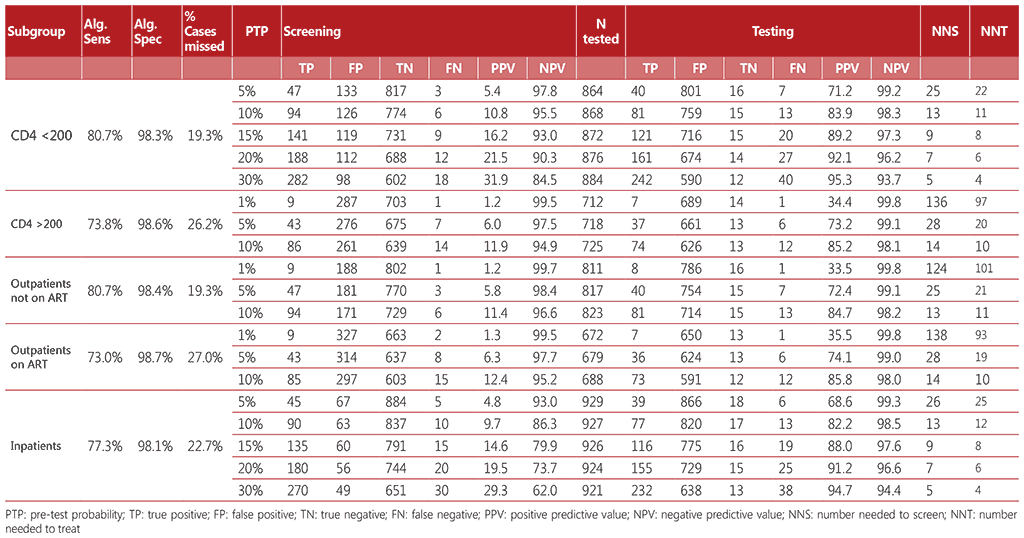
Table A2.3 Algorithm 3
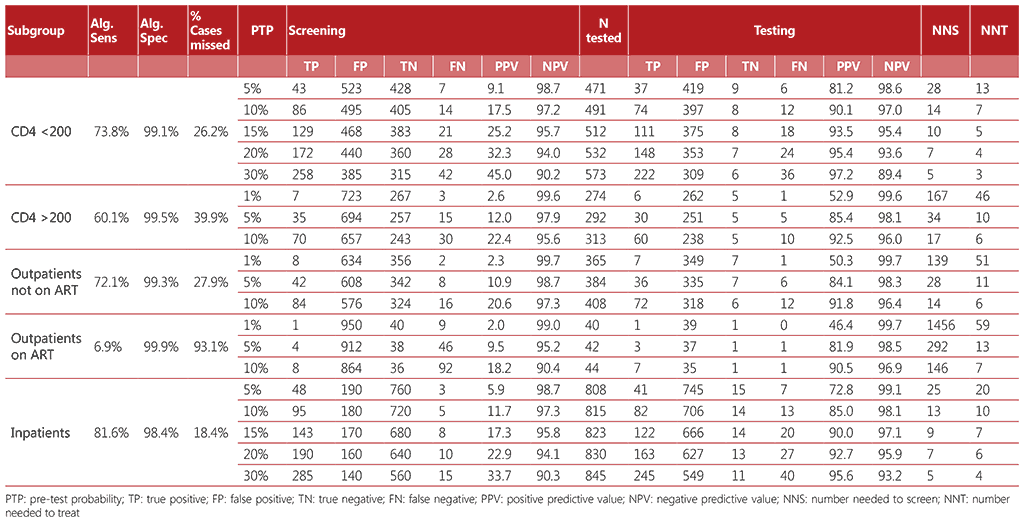
Table A2.4 Algorithm 4
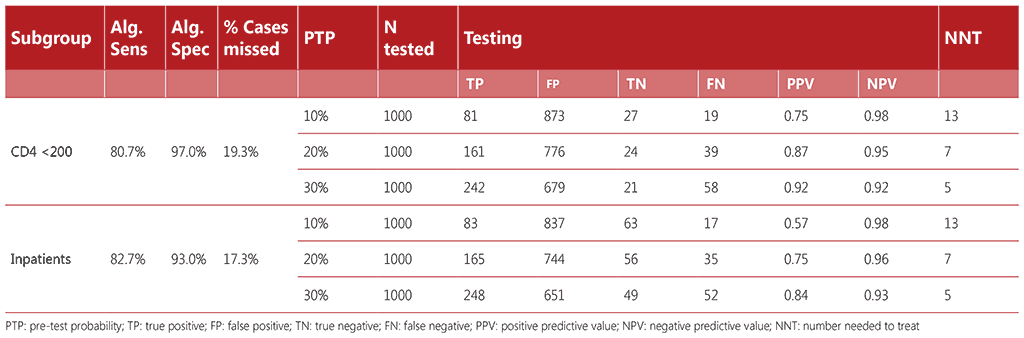
Table A2.5 Cost estimates (median costs)
Costs associated with screening 1000 people with HIV (PTP 1%)
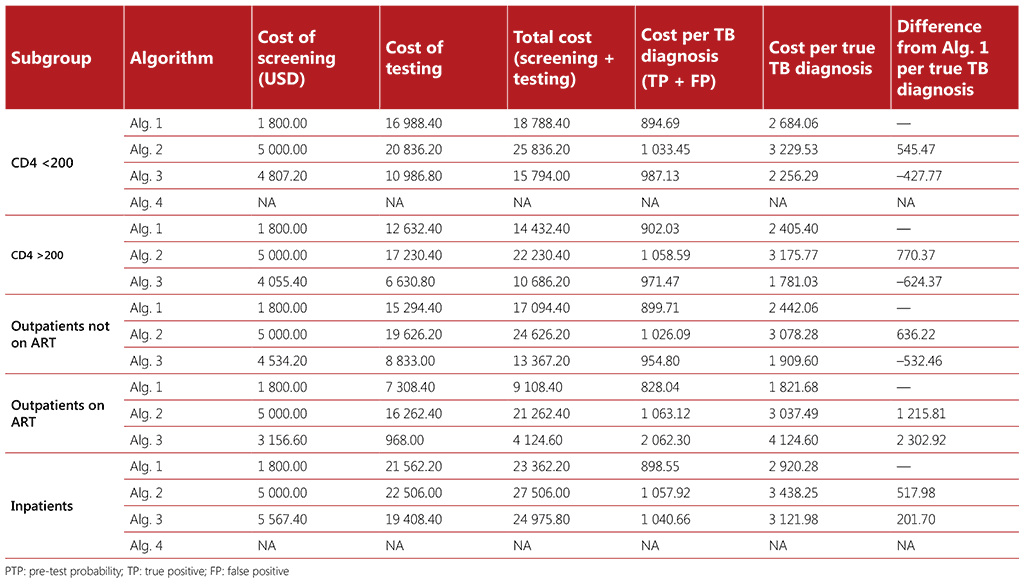
Table A2.6 Cost estimates (median costs)
Costs associated with screening 1000 people with HIV (PTP 10%)
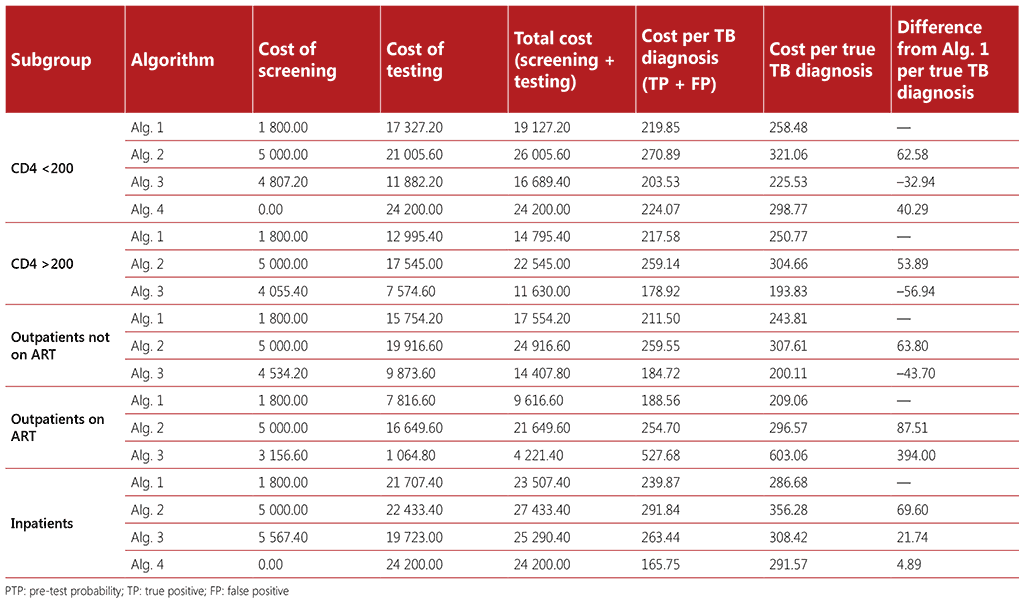
References for Annex 2
- World Health Organization. WHO operational handbook on tuberculosis. Module 2: screening - systematic screening for tuberculosis disease. Geneva; 2020 (9789240001503; https://apps.who.int/iris/handle/10665/340256.
- World Health Organization. WHO consolidated guidelines on tuberculosis Module 2: Screening – Systematic screening for tuberculosis disease. Geneva 2021 (https://iris.who.int/handle/10665/340255.
- Dhana A, Hamada Y, Kengne AP, Kerkhoff AD, Rangaka MX, Kredo T et al. Tuberculosis screening among ambulatory people living with HIV: a systematic review and individual participant data meta-analysis. Lancet Infect Dis. 2022;22:507-18. doi: 10.1016/S1473-3099(21)00387-X.
- Dhana A, Hamada Y, Kengne AP, Kerkhoff AD, Rangaka MX, Kredo T et al. Tuberculosis screening among HIV-positive inpatients: a systematic review and individual participant data meta-analysis. Lancet HIV. 2022;9:e233-e41. doi: 10.1016/S2352-3018(22)00002-9.
- WHO consolidated guidelines on tuberculosis. Module 3: diagnosis - rapid diagnostics for tuberculosis detection, 2021 update. Geneva: World Health Organization; 2021 (https://apps.who.int/iris/handle/10665/342331, accessed 31 August 2023).
- WHO operational handbook on tuberculosis. Module 3: diagnosis - rapid diagnostics for tuberculosis detection, 2021 update. Geneva: World Health Organization; 2021 (https://apps.who.int/iris/handle/10665/342369, accessed 31 August 2023).
- Horne DJ, Kohli M, Zifodya JS, Schiller I, Dendukuri N, Tollefson D et al. Xpert MTB/RIF and Xpert MTB/ RIF Ultra for pulmonary tuberculosis and rifampicin resistance in adults. Cochrane Database Syst Rev. 2019;6:CD009593. doi: 10.1002/14651858.CD009593.pub4.
- Yoon C, Semitala FC, Asege L, Katende J, Mwebe S, Andama AO et al. Yield and Efficiency of Novel Intensified Tuberculosis Case-Finding Algorithms for People Living with HIV. Am J Respir Crit Care Med. 2019;199:643- 50. doi: 10.1164/rccm.201803-0490OC.
- Shah M, Ssengooba W, Armstrong D, Nakiyingi L, Holshouser M, Ellner JJ et al. Comparative performance of urinary lipoarabinomannan assays and Xpert MTB/RIF in HIV-infected individuals. AIDS. 2014;28:1307-14. doi: 10.1097/QAD.0000000000000264.
- Peter J, Theron G, Chanda D, Clowes P, Rachow A, Lesosky M et al. Test characteristics and potential impact of the urine LAM lateral flow assay in HIV-infected outpatients under investigation for TB and able to selfexpectorate sputum for diagnostic testing. BMC Infect Dis. 2015;15:262. doi: 10.1186/s12879-015-0967-z.
- Andama A, Jaganath D, Crowder R, Asege L, Nakaye M, Katumba D et al. Accuracy and incremental yield of urine Xpert MTB/RIF Ultra versus Determine TB-LAM for diagnosis of pulmonary tuberculosis. Diagn Microbiol Infect Dis. 2020;96:114892. doi: 10.1016/j.diagmicrobio.2019.114892.
- Sweeney S, Laurence YV, Cunnama L, Gomez GB, Garcia-Baena I, Bhide P et al. Cost of TB services: approach and summary findings of a multi-country study (Value TB). Int J Tuberc Lung Dis. 2022;26:1006-15. doi: 10.5588/ijtld.22.0096.
- Capeding TPJ, Rosa JD, Lam H, Gaviola DG, Garfin AMC, Hontiveros C et al. Cost of TB prevention and treatment in the Philippines in 2017. Int J Tuberc Lung Dis. 2022;26:392-8. doi: 10.5588/ijtld.21.0622.
- Chatterjee S, Toshniwal MN, Bhide P, Sachdeva KS, Rao R, Laurence YV et al. Costs of TB services in India (No 1). Int J Tuberc Lung Dis. 2021;25:1013-8. doi: 10.5588/ijtld.21.0105.
- Chikovani I, Shengelia N, Marjanishvili N, Gabunia T, Khonelidze I, Cunnama L et al. Cost of TB services in the public and private sectors in Georgia (No 2). Int J Tuberc Lung Dis. 2021;25:1019-27. doi: 10.5588/ ijtld.21.0176.
- Kairu A, Orangi S, Oyando R, Kabia E, Nguhiu P, Ong Ang O J et al. Cost of TB services in healthcare facilities in Kenya (No 3). Int J Tuberc Lung Dis. 2021;25:1028-34. doi: 10.5588/ijtld.21.0129.
- Bjerrum S, Schiller I, Dendukuri N, Kohli M, Nathavitharana RR, Zwerling AA et al. Lateral flow urine lipoarabinomannan assay for detecting active tuberculosis in people living with HIV. The Cochrane database of systematic reviews. 2019;10:CD011420-CD. doi: 10.1002/14651858.CD011420.pub3.